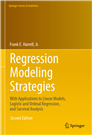
ISBN
| 9783319194240 |
DDC
| 519.536 |
Tác giả CN
| Harrell, Frank E. |
Nhan đề
| Regression modeling strategies : with applications to linear models, logistic regression, and survival analysis / Frank E Harrell |
Lần xuất bản
| Second edition |
Thông tin xuất bản
| New York : Springer, 2015 |
Mô tả vật lý
| 598 pages. : illustrations |
Tóm tắt
| This highly anticipated second edition features new chapters and sections, 225 new references, and comprehensive R software. In keeping with the previous edition, this book is about the art and science of data analysis and predictive modeling, which entails choosing and using multiple tools. Instead of presenting isolated techniques, this text emphasizes problem solving strategies that address the many issues arising when developing multivariable models using real data and not standard textbook examples. It includes imputation methods for dealing with missing data effectively, methods for fitting nonlinear relationships and for making the estimation of transformations a formal part of the modeling process, methods for dealing with "too many variables to analyze and not enough observations," and powerful model validation techniques based on the bootstrap.℗ℓ The reader will gain a keen understanding of predictive accuracy, and the harm of categorizing continuous predictors or outcomes.℗ℓ This text realistically deals with model uncertainty, and its effects on inference, to achieve "safe data mining." It also presents many graphical methods for communicating complex regression models to non-statisticians. Regression Modeling Strategies presents full-scale case studies of non-trivial datasets instead of over-simplified illustrations of each method. These case studies use freely available R functions that make the multiple imputation, model building, validation, and interpretation tasks described in the book relatively easy to do. Most of the methods in this text apply to all regression models, but special emphasis is given to multiple regression using generalized least squares for longitudinal data, the binary logistic model, models for ordinal responses, parametric survival regression models, and the Cox semiparametric survival model.℗ℓ A new emphasis is given to the robust analysis of continuous dependent variables using ordinal regression. As in the first edition, this text is intended for Masters' or Ph. D. level graduate students who have had a general introductory probability and statistics course and who are well versed in ordinary multiple regression and intermediate algebra. The book will also serve as a reference for data analysts and statistical methodologists, as it contains an up-to-date survey and bibliography of modern statistical modeling techniques. Examples used in the text mostly come from biomedical research, but the methods are applicable anywhere predictive models ("analytics") are useful, including economics, epidemiology, sociology, psychology, engineering, and marketing. |
Thuật ngữ chủ đề
| Statistics |
Thuật ngữ chủ đề
| Regression analysis |
Thuật ngữ chủ đề
| Linear models (Statistics) |
Thuật ngữ chủ đề
| Mathematical statistics |
Khoa
| Khoa Cơ bản |
Địa chỉ
| Thư Viện Đại học Nguyễn Tất Thành |
|
000
| 00000nam#a2200000u##4500 |
---|
001 | 21045 |
---|
002 | 2 |
---|
004 | 4268E44F-ED7D-4AE4-96AD-10AB3BB5D725 |
---|
005 | 202006041515 |
---|
008 | 200514s2015 nyu eng |
---|
009 | 1 0 |
---|
020 | |a9783319194240 |
---|
039 | |a20200604151537|btainguyendientu|y20200514090403|zquyennt |
---|
040 | |aNTT |
---|
041 | |aeng |
---|
044 | |anyu |
---|
082 | |a519.536|bH2966|223 |
---|
100 | |aHarrell, Frank E. |
---|
245 | |aRegression modeling strategies : |bwith applications to linear models, logistic regression, and survival analysis / |cFrank E Harrell |
---|
250 | |aSecond edition |
---|
260 | |aNew York : |bSpringer, |c2015 |
---|
300 | |a598 pages. : |billustrations |
---|
504 | |a
Includes bibliographical references (pages 555-586) and index.
|
---|
520 | |aThis highly anticipated second edition features new chapters and sections, 225 new references, and comprehensive R software. In keeping with the previous edition, this book is about the art and science of data analysis and predictive modeling, which entails choosing and using multiple tools. Instead of presenting isolated techniques, this text emphasizes problem solving strategies that address the many issues arising when developing multivariable models using real data and not standard textbook examples. It includes imputation methods for dealing with missing data effectively, methods for fitting nonlinear relationships and for making the estimation of transformations a formal part of the modeling process, methods for dealing with "too many variables to analyze and not enough observations," and powerful model validation techniques based on the bootstrap.℗ℓ The reader will gain a keen understanding of predictive accuracy, and the harm of categorizing continuous predictors or outcomes.℗ℓ This text realistically deals with model uncertainty, and its effects on inference, to achieve "safe data mining." It also presents many graphical methods for communicating complex regression models to non-statisticians. Regression Modeling Strategies presents full-scale case studies of non-trivial datasets instead of over-simplified illustrations of each method. These case studies use freely available R functions that make the multiple imputation, model building, validation, and interpretation tasks described in the book relatively easy to do. Most of the methods in this text apply to all regression models, but special emphasis is given to multiple regression using generalized least squares for longitudinal data, the binary logistic model, models for ordinal responses, parametric survival regression models, and the Cox semiparametric survival model.℗ℓ A new emphasis is given to the robust analysis of continuous dependent variables using ordinal regression. As in the first edition, this text is intended for Masters' or Ph. D. level graduate students who have had a general introductory probability and statistics course and who are well versed in ordinary multiple regression and intermediate algebra. The book will also serve as a reference for data analysts and statistical methodologists, as it contains an up-to-date survey and bibliography of modern statistical modeling techniques. Examples used in the text mostly come from biomedical research, but the methods are applicable anywhere predictive models ("analytics") are useful, including economics, epidemiology, sociology, psychology, engineering, and marketing. |
---|
541 | |aSpringer |
---|
650 | |aStatistics |
---|
650 | |aRegression analysis |
---|
650 | |aLinear models (Statistics) |
---|
650 | |aMathematical statistics |
---|
690 | |aKhoa Cơ bản |
---|
852 | |aThư Viện Đại học Nguyễn Tất Thành |
---|
856 | 1|uhttp://elib.ntt.edu.vn/documentdata01/2 tailieuthamkhao/500 khoahoc/anhbiasach/21045_regressionmodelingstrategiesthumbimage.jpg |
---|
890 | |c1|a0|b0|d2 |
---|
| |
Không tìm thấy biểu ghi nào
|
|
|
|